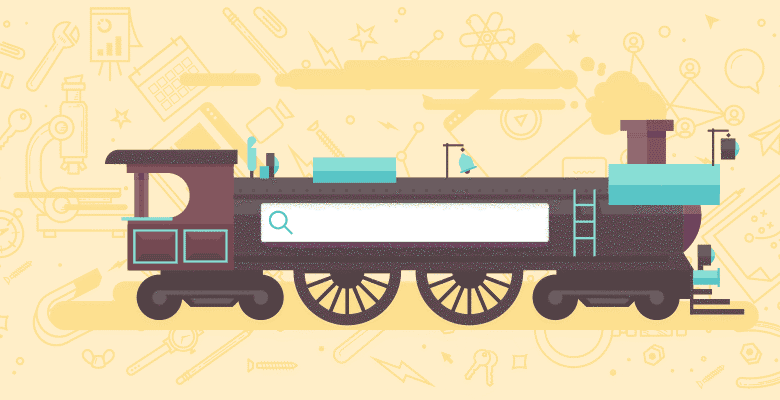
(NOTE: This post is written by Ben Hendrickson and Rand Fishkin as a follow up to Ben’s presentation at the Distilled/SEOmoz training seminar in London this week)
Our web index, Linkscape, updated again recently, and in addition to provide the traditional stats, we thought we’d share some of the cutting edge research work we do here. Below, you’ll find a post which requires extremely close and careful reading. Correlation data doesn’t have all the answers, but it’s certainly very interesting. Likewise, the ranking models data provides a great deal of insight, but it would be dangerous to simply look at the charts without reading the post carefully. There’s a number of caveats and information – raw lines can mislead by themselves, so please be diligent!
[UPDATE Oct 26, 2009: There used to be a mistake. Below there is a line showing showing correlation between unique domains linking and SERP order. That line was previously incorrect. Instead of being the number of unique domains linking to the target page, it was the number of unique domains linking anywhere on the domain of the target page. The corrected line shows unique domains linking to be much more important, so I also added this line to the combined über score vs individual features chart. My apologies for the error. -Ben]First, some stats on the latest Linkscape index:
- Release date: Oct. 6th, 2009 (exactly 1 year after our initial launch)
- Root Domains: 57,422,144 (57 million)
- Subdomains: 215,675,235 (215 million)
- URLs: 40,596,773,936 (40.5 billion)
- Links: 456,939,586,207 (456 billion; if we also include pages that 301, that number climbs to 461 billion)
- Link Attributes:
- No-follow link, internal: 6,965,314,198 (1.51% of total)
- No-follow links, external: 2,765,319,261 (0.60% of total)
- No-follow links, total: 9,730,633,459 (2.11% of total)
- 301’ing URLs: 384,092,425 (0.08% of total)
- 302’ing URLs: 2,721,705,173 (0.59% of total)
- URLs employing ‘rel=canonical’: 52,148,989 (0.01% of total)
- Average Correlation between mozRank + Google PageRank
- Mean absolute error: 0.54
- Average Correlation between Domain mozRank (DmR) and Homepage PageRank
- Mean absolute error: 0.37
Now let’s get into some of the research around correlation data and talk about how we can use the features Linkscape provides to produce some interesting data. These first charts use raw correlation – just the relationship between the ranking positions and the individual feature. As noted above, please read the descriptions of each carefully before drawing conclusions and remember that correlation IS NOT causation. These charts are not meant to say that if you do these things, you will instantly get better rankings – they merely show what features apply to pages/sites ranking in the top positions.
Understanding the Charts:
- Mean Index By Value: These are used for the y-axises of many charts. Instead of averaging the raw values, we average it’s relative index in the SERP if ordered by this value. So if there are 3 SERPs, and the page in the first position has the 4th most links, the 2nd has the 1st most links, and the 3rd has the 10th most links, the mean index by # of links for the first position would be (4+1+10)/3 = 5.
- Mean Count Numbers – these numbers appear on the y-axis of the first chart, showing averages of link counts.
- Position: This is used for the x-axises of many charts. For these charts, this is specific to the organic position in Google.com, excluding any vertical or non-traditional search results (local, video, news, images, etc.)
- Error Bars: The bars that bound the trend lines in our charts can show the confidence of two different things. On some charts, they show the 95% of confidence of where the mean will be if we had infinite analogous data. These error bars show how confident we are in the line, and frequently have the word “stderr” in the title. On other charts, they are our confidence of what any given SERP will look like. These error bars are much wider, as we are much more certain of what the average of many SERPs will be than we are what any given SERP will look like. Charts with these error bars are frequently labeled with “stddev” in the title.
The data below is based on a collection of 10,000 search results for a variety of queries (biased towards generic and commercial rather than branded/informational queries) and 250,000 results. Some results were excluded for errors during crawling or returning non-html responses. Results are taken from Google.com in the US from October of 2009.
Are Links Well Correlated with Rankings?
Common SEO wisdom holds that the raw number of links that points to a result is a good predictor of ranking position. However, many SEOs have noticed that Yahoo! Site Explorer’s link numbers (and even Google’s numbers inside services like Webmaster Tools) can include a dramatic number of links that may not matter (nofollowed links, internal links, etc.) and exclude things (like 301 redirects) that matter quite a bit. Using the Linkscape data set, we can remove these noisy links and use only the number of external, followed links (and 301s) to run in our correlation analysis.
This first chart certainly suggests that correlation exists, but the spikiness is a bit frustrating. Through deeper analysis, we found that this is largely due to results that have ranking pages with massive (or very tiny) quantities of links. Thus, it made sense to produce this next chart:
Here, we can see what would happen if we force-rank the results by number of links. This means we’ve taken each set of results and assigned a number (1, 2, 3, etc.) that corresponds to the quantity of links they have in comparison to the other pages ranking for that result (e.g. the page with the most links is assigned “1,” the second-most links gets “2,” etc). The smoothness of the line suggests it is fairly accurate, but we can be precise about our accuracy. The error bars below show the 95th percentile confidence interval for estimates of the mean.
We’re looking good. The correlation is quite strong, suggesting that yes, the number of external, followed links is important and the standard error is low, so we can feel confident that the correlation is real. Clearly, though, comparing with the perfect fit line, links are not the whole picture. Having the most links out of your peers in the results is likely a very good goal, but it can’t be the only goal.
The last piece here is to examine the standard deviation. This can tell us how much an individual page might vary from the averages.
This chart tells us that variation for any individual set of results can be quite large, so getting more links isn’t always going to be a clear win. It’s notable that in this chart, standard deviation here is shown for the 95th percentile confidence, which is actually 1.97 standard deviations away from the mean. On the whole, # of external, followed links is clearly important and well correlated, but we’re going to need to get more advanced in our models and broader in our thinking to get actionable information at a granular level.
Can Any Single Metric Predict the Rankings?
Boy, that sure would be nice… We’ve looked in the past at the quality of metrics like PageRank, Yahoo! Site Explorer’s Link Counts, Alexa Rank, etc. The short answer is that they’re barely better than random guessing. Google’s PageRank score was (around February of 2009) approximately 16% better than random guessing for predicting ranking page (N+10 aka ranking page 1 vs. page 2) and less than 5% better than random guessing for predicting ranking position (N+1 aka ranking position 1 vs position 2). The chart below shows correlations for a number of popular SEO metrics:
Since then, Nick, Ben and Chas have all been hard at work on improving the value and quality of Linkscape’s index as well as the usefulness and signaling provided by the metrics. This next chart shows how we’re progressing:
The correlations above map in the 35-50% better than random guessing range (though it’s not a 1-to-1 comparison with the numbers above – watch for that in a future post) for the first result. Looking at this graph suggests that external mozRank (which represents the quantity of link juice to a page from external links) and external followed links correspond well to current rankings is interesting and certainly lends an additional data point for link builders. This correlation line might, for example, suggest that in the “average” rankings scenario, earning links from high mozRank/PageRank pages with few links on them (so the links pass more juice) as well as higher raw quantities of external, followed links are both very important. But even more, this chart supports the idea that earning links from unique domains is paramount.
[UPDATE (Oct 26, 2009): Previously there was a paragraph speculating why the above result for the importance of unique linking domains was so much lower than we previously calculated. As noted at the top of this post, this was because I used the wrong datapoint for unique domains linking. Correcting this made the discrepancy with earlier results disappear. The chart above is now correct. -Ben]The frustrating part about this data is that it’s not telling us the entire story, nor is it directly actionable for an individual search query. As you can see below, the standard deviation numbers show that for any given search, the range varies somewhat dramatically.
When we see this effect, just as we did above, takeaway for an SEO doing work on a client project and attempting to achieve a particular ranking position is unclear. Employing these metrics as KPIs and ways of valuing potential links is probably useful, and building competitive analysis tracking with these data points is likely to be considerably better than using more classic third-party metrics, but it doesn’t say “do this to rank better,” and that’s the “holy grail” we’re chasing.
How Do “On-Page” Factors Correlate with Rankings?
This post has dealt very little with on-page factors and their correlation to rankings. We’ll look at that next.
Google recently announced that they ignore the meta keywords tag. This data, showing a line that’s very spiky and error bars showing stderr (standard error) all within the horizontal at “13” certainly supports that assertion. Employing the query term/phrase in the meta keywords is one of the least correlated signals we’ve examined.
Title tags that employ the query term, on the other hand, appear to have a real correlation with rankings. They’re certainly not perfectly correlated, but on average, this chart tells us that Google has a clear preference (though not massively strong – note the smaller range in the y-axis) for pages that employ the query term in the title tag.
We’ve examined H1/H2/Hx tags in the past and come to the conclusion that they have little impact on rankings. This graph certainly suggests that’s still the case. Employing the query in other on-page areas such as the body (anything between the
tags) and out anchors (employing the keyword in the tag whether internal or external) have significantly greater correlation with rankings, while H1-H4 tag keyword use appears almost horizontal on the graph (suggesting no benefit is derived from its use). It’s not as bad as the random effect we observed with meta keywords (the lines all start a tiny bit below 13 and end a tiny bit above), but the positive correlation is low and the horizontal is mostly inside the error bars.This graph is the clearest illustration yet of why it’s so important to build systems more advanced than simple, direct correlation. According to this chart, employing the query term in the path or filename of the URL is actually slightly negatively correlated with ranking highly, while the subdomain appears largely useless and the root domain has strong correlation. Granted, all of these (except the root domain) are on a very narrow band of the x-axis, but SEO experience tells us that using keywords in the name of a page is a very good thing, for both search rankings and click-through rate. Whenever we see data like this, a number of hypotheses arise. The one we like best internally right now is that the URL path/filename data may be skewed by the root domain keyword usage. Essentially, when a root domain name already employs the keyword term, the engines may see those who also employ it in the path/filename as potentially keyword stuffing (a form of spam). It may also be that raw correlation sees a large number of less-well URL-optimized pages performing well due to other factors (links, domain authority, etc.). It’s also true that most sites that employ the keyword in the path/filename don’t use it in the root domain as well, so the negative of the one may be mixed-in with the positive of the other.
Whatever the reason, this is a perfect example of why raw correlation is flawed and why a greater depth of analysis – and much more sophisticated models – are critical to getting more value out of the data.
Can We Build a Ranking Model that Gives more Actionable Takeaways?
To get to a true representation of the potential value of any given SEO action, we need a model that imitates Google’s. This is no easy undertaking – Google employs a supposed 200 ranking factors, so while we’ve got lots of data points (on-page and link factors, plus lots of derivatives/combinations of these) the complexity is still a dramatic hurdle.
The “uber” score (red line in the graph above) is built by taking all of these features we have about webpages, domains and links from both on-page analysis and Linkscape data. We (well, technically, Ben) run them through a machine learning model that maps to the search results and produces a result that’s considerably better correlated with rankings than any single metric. You can already see that in the top 10 search results, the slope of the line is looking really good – an indication that our metrics and analysis function better for predicting success in those areas (which, luckily, are the same positions SEOs care most about).
These machine learning ranking models let us take a much more sophisticated look at the value of employing a keyword in any particular on-page feature. Instead of going off simple correlation, we can actually ask, based on our best fit model, “what’s the impact of using the keyword here?” Let’s use the example we struggled with above showing negative correlation for keywords in path/filename:
As you can see, this model suggests that, once again, subdomains are largely useless places to put keywords, but the root domain is a very good place to employ it. Path and filename are slightly positive, which also fits with our expectations. It’s also important to note that on this chart some lines dip below 0 on the “mean derivative of uber” y-axis in the 20-25 ranking position range. This suggests that for those results, the keyword use may actually be hurting them. Looking into some sample results, we can see that a number of the URLs in that 20-25 range seem to be trying too hard. They’re using the keyword multiple times in the domain/path/filename and fit with what many SEOs call “spammy-looking.” It could certainly be a weakness in our model’s accuracy, but we think it’s also likely that a lot of pages would actually benefit from being a bit less aggressive with their URL keyword stuffing.
In this next chart, we can see the standard deviation error bars. You can see that we’re more confident that in the top results, employing keywords in these URL features won’t hurt and is likely to help, while in the latter portion of the results, we’ve got a bit less confidence about the negative effects.
Let’s turn our attention to those pesky H(x) tags again, and see if the ranking model has more to say about their impact/value.
We’re still getting mostly similar results. It appears that H1-H4 tags are not great places to use keywords. As with the URL features, they seem to help a tiny bit (even less than URL features, actually), then have a very tiny negative – flat effect in the latter SERPs. Even with the error bars, this is fairly convincing evidence that H(x) tags just don’t provide much value. A best practice might still suggest their use, but there are probably far more valuable places to use your keywords.
Our link measurements also get more sophisticated (and tell a more nuanced story) when we use the ranking models. You can see above that improving mozRank in the top results appears important, while raw # of links may be less valuable. However, when we look further back in the results, you see the negative dip, suggesting that some pages may be over-using mozRank and external links (quite possibly from less reputable/spammy sources). This graph doesn’t have a ton of actionable data (as controlling the amount of mozRank or even the number of external links you get is probably not wise), but it does fit fairly nicely to a lot of the things we know about SEO – good links help, bad links might hurt.
The last graph shows some of the more interesting on-page features from our dataset. The big one here is the consistent suggestion to use images with good alt text that employ your keyword term/phrase. That green line is one of our highest correlations for on-page keyword usage. Putting keywords in bold, in body text (anywhere) and even in out anchors (remember, these are any anchors, not necessarily external links) has the same type of positive impact at top SERPs and slight negative in the 20-25 range that we’ve seen previously. This shouldn’t surprise us at all is we suspect that spammers/keyword-stuffers are playing more heavily in those result numbers.
Conclusions & Take-Aways:
I know this is a lot of data to parse, but it’s also pretty important to understand if you’re in the SEO space and want to bring more data credibility and analysis to your projects. We suspect that SEOmoz isn’t the only firm working on this (though we may be the only one willing to publicly share the data for now), and you can bring a lot of credibility to a client project or in-house effort with these data points showing the importance and predicted value of the changes you recommend as an SEO. There are plenty of people who malign our industry as being based on hunches and intuition rather than strong data. With these analyses, we’re getting closer to closing that gap. We don’t want to suggest that this data is perfect (the error bars and accuracy analyses show that’s obviously not the case), but it’s certainly a great extra piece to add to the equation.
Things the data suggests that we feel good about:
- Links are important, but naive link data can mislead. It seems wise to get more sophisticated with link analysis.
- No single metric can predict rankings (at least, not yet)
- H1s (and H2s-H4s) probably aren’t very important places to use your keywords
- Alt attributes of images are probably pretty important places to use your keywords
- Keyword stuffing may be holding you back (particularly if you’re outside the top 15 results and overusing it)
- Likewise, overdoing it with (not-so-great) links might be hurting you
We’re definitely looking forward to comments and questions, but Ben & I are in the UK and may not be back online for a while (Ben’s plane leaves in a few hours for the US and British Airways doesn’t yet have wifi in-flight).
p.s. A shoutout to Tim Grice from SEOWizz, who put together this correlation analysis a few weeks back.